Ongoing reseach projects
Questions driving my PhD study are:
What processes (especially physiologically) are critical for trees under extreme conditions,
and how can we accurately capture/represent them in our models? The overall aim is to make better projections and increase the understanding of how trees respond to climate change.
Modelling plant water storage and its influences on tree’s growth and functioning under drought
This study will use the data from the experiment (short-term and long-term drought) forest site Skogaby (South Sweden), and it will calibrate based on soil water and tree biomass, nutrient data. The aim is to invesitage the impacts of drought on caron sequestration and physiological processes; further develop and validate CoupModel that captures the intricate dynamics of tree's responses to drought conditions (Precipitation was experimentally reduced by ~70% during the growing seasons 1990-1997). We use CoupModel v6.0, also called Coup-CNP. This version explicitly integrates the P cycle and root-mycorrhiza fungi symbiosis.
A schematic digram of CoupModel components:
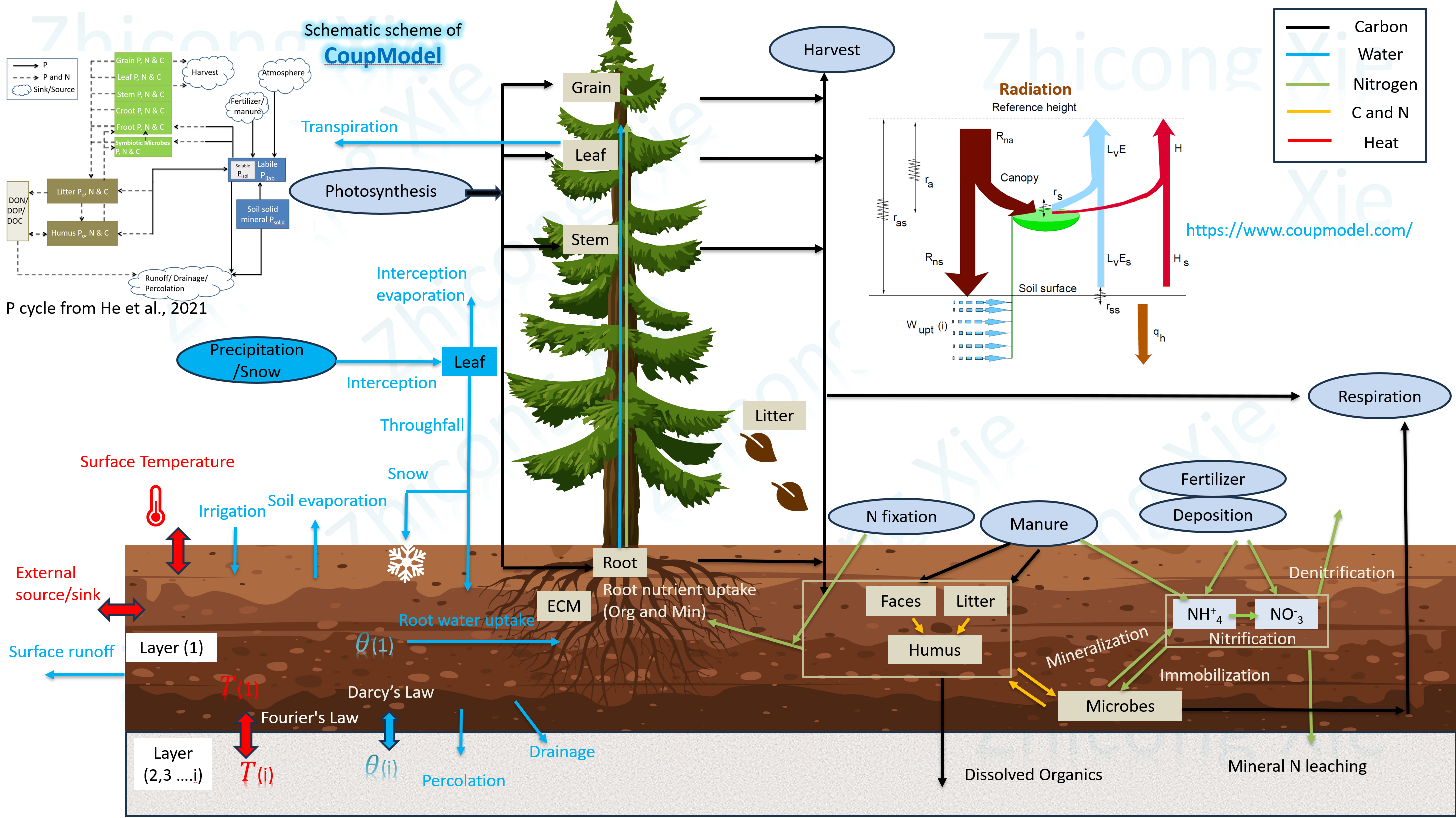
Regional modelling (planning)
A dynamic vegetation model, LPJGUESS, will be used for future scaling-up, extending its study area to cover a wider range of ecosystems. Planning topics are:
1. Compare different scales (Bridging CoupModel and LPJGUESS?);
2. Forest management scenarios (future scenarios in monoculture and mixed forest);
3. Climate-vegetation feedbacks at regional scale.
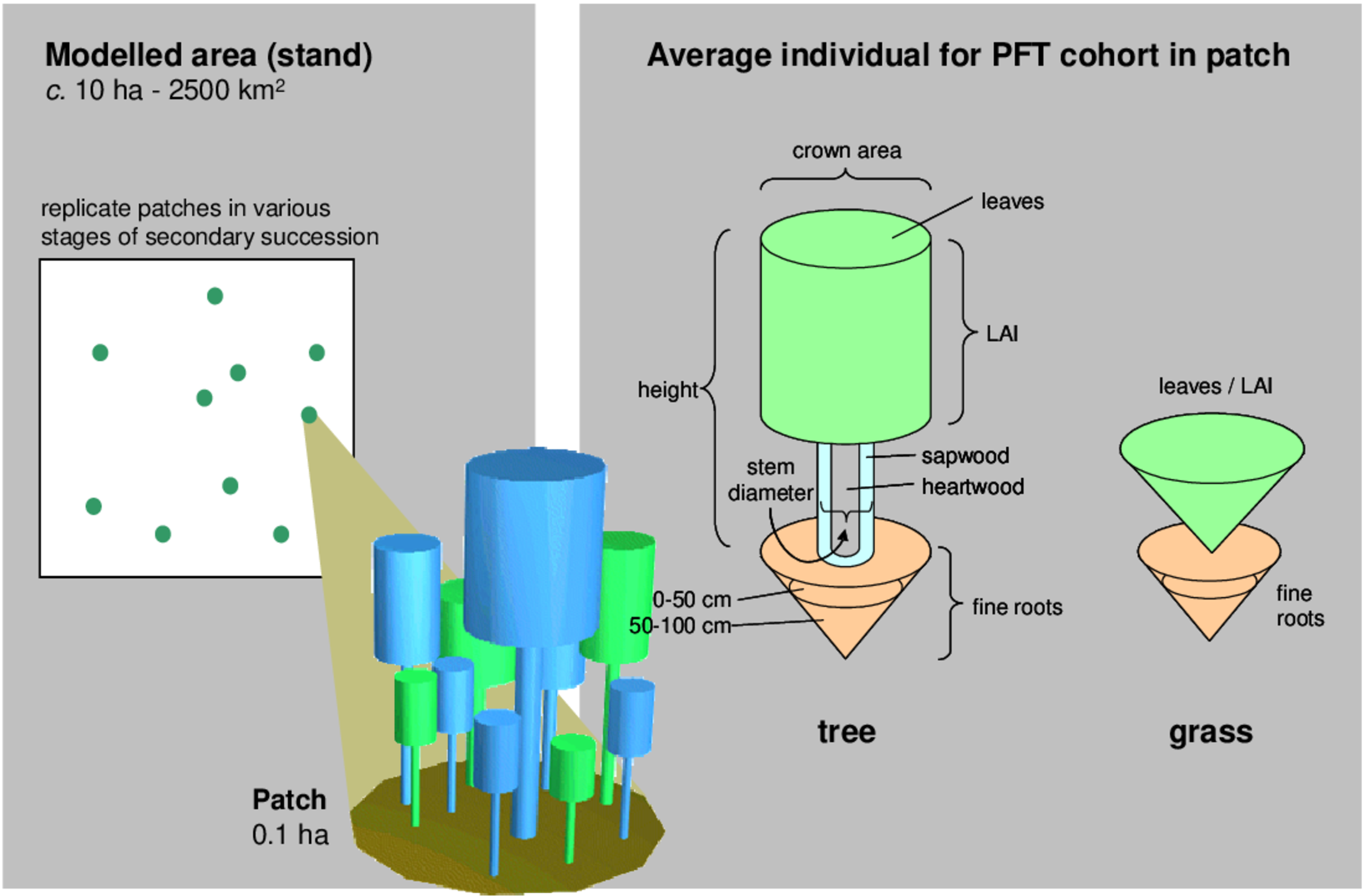
Ben Smith (2007)
Urban tree modelling (planning)
CoupMmdel will also be employed in an urban tree project, enabling the forecasting of both current and future implications arising from climate stress factors such as drought,
heatwaves, and flooding. This study will specifically focus on diverse tree species and environments, including parks and traffic lanes, within the city of Gothenburg. High-resolution satellite and remote sensing data will also be used to explore the city's greenery. These datasets serve as complementary information, enhancing our ability to interpret and interpolate results.
With their high resolution in spatial, spectral, and temporal dimensions, a machine learning method will be used to predict and assess urban vegetation vulnerability to drought, it also allows us to identify the importance of different factors, help analyze various tree characteristics and understand how different factors—such as weather, soil conditions, and the trees themselves—impact their extreme conditions tolerance.
Temperature-Vegetation Drought Index (TVDI) is used as a proxy indicator that represents the moisture conditions.
Utilizing the spatial correlation between land surface temperature (LST) and the NDVI as indicators for drought and soil moisture conditions. TVDI was considered suitable for predicting short-term droughts during the growing season.
Calculated TVDI in a drought year 2018:
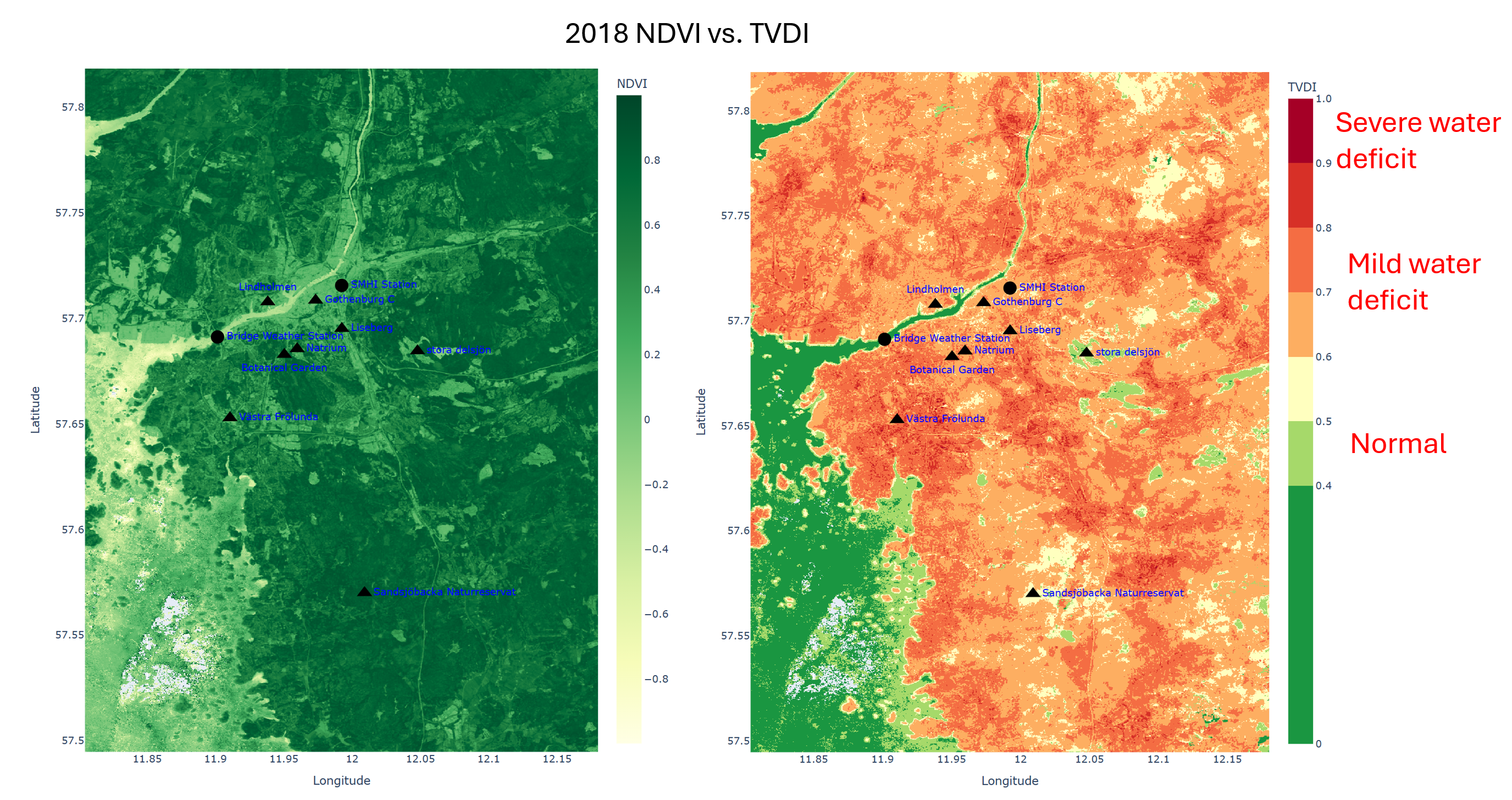

Other topics......
I am also interested in spatial-temporal 'big data' analysis from observational, reanlysis data, outputs from Earth system models (ESMs), and remote sensing data.
These diverse source are used to explore the impacts of climate change on the carbon cycle, vegetation dynamics, and ecosystem services. I am excited to use multi source-data, and integrate modelling,
with observational and experimental methods, to uncover the 'something' occurring beneath ecosystem as the damn climate change is hapenning and getting worse
(2024 is on track to be hottest year on record as warming temporarily hits 1.5°C)
Feel free to reach out if you share common interests and would like to talk. Hope I will not end up like this 😂:
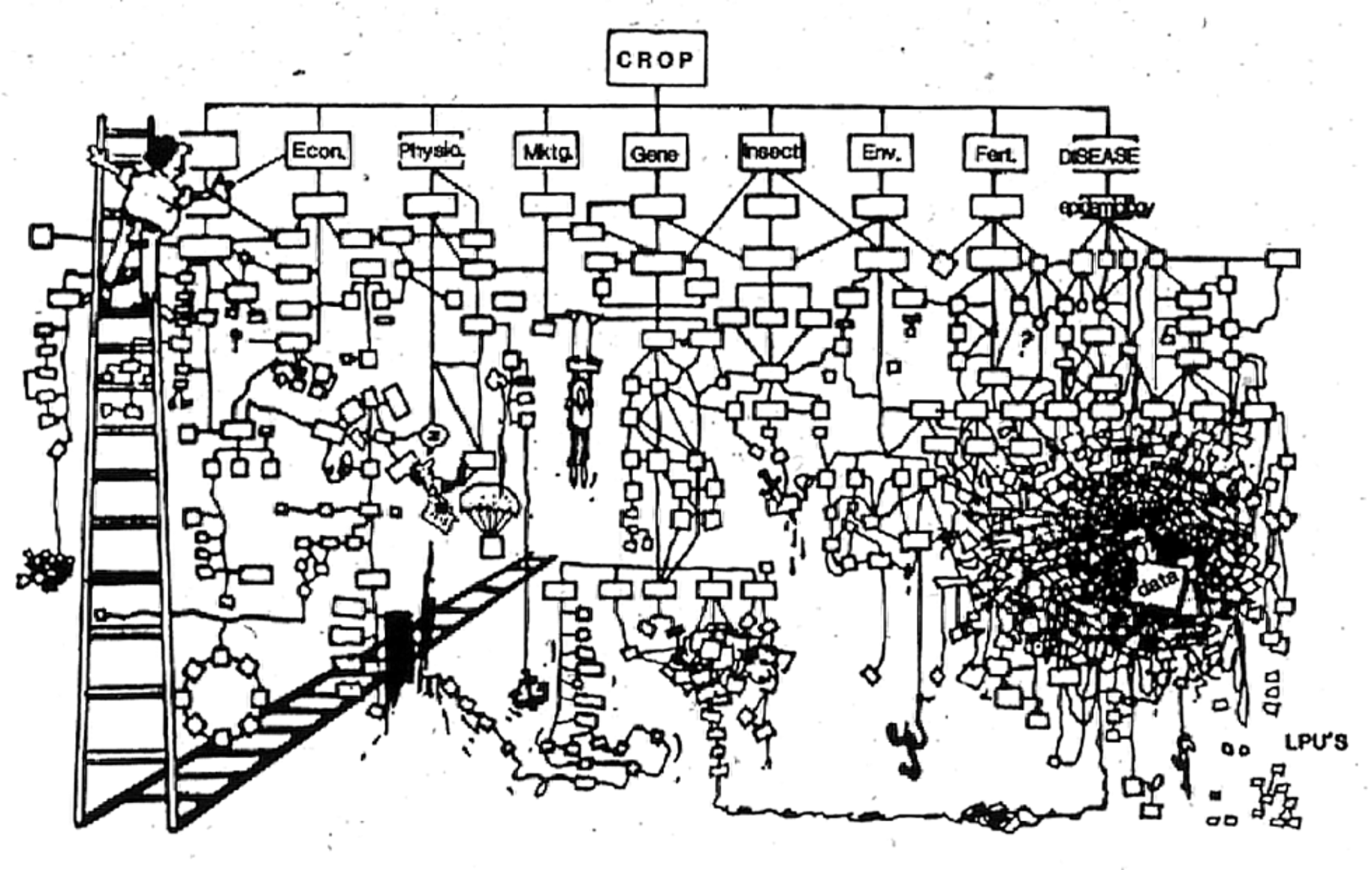
Marster's research
Click here to the thesis
The Arctic is experiencing a phenomenon called Arctic amplification (AA) which has caused a rapid warming rate two to three times greater than that of the Northern hemisphere (NH) or global in both observed and modelled data over the past few decades. Previous studies focused mostly on temperature and sea ice albedo feedbacks; however, the impacts of biosphere feedbacks especially from carbon cycle feedbacks, are largely underexplored. In this study, different coupling experiments simulated by 11 Earth system models (ESMs) were used to assess the spatial and temporal changes of AA, as well as to identify potential causes related to carbon cycle feedbacks.
In this study, we aim to answer the following questions:
1. What are the spatial-temporal patterns of AA in different C4MIP experiments? What are the AA changes resulting from carbon-concentration feedback and carbon-climate feedback?);
2. Are these patterns consistent or inconsistent among Earth system models?;
3. If there are synergic or counteracting effects from carbon-concentration and carbon-climate feedbacks, are these effects associated with ocean or land processes?
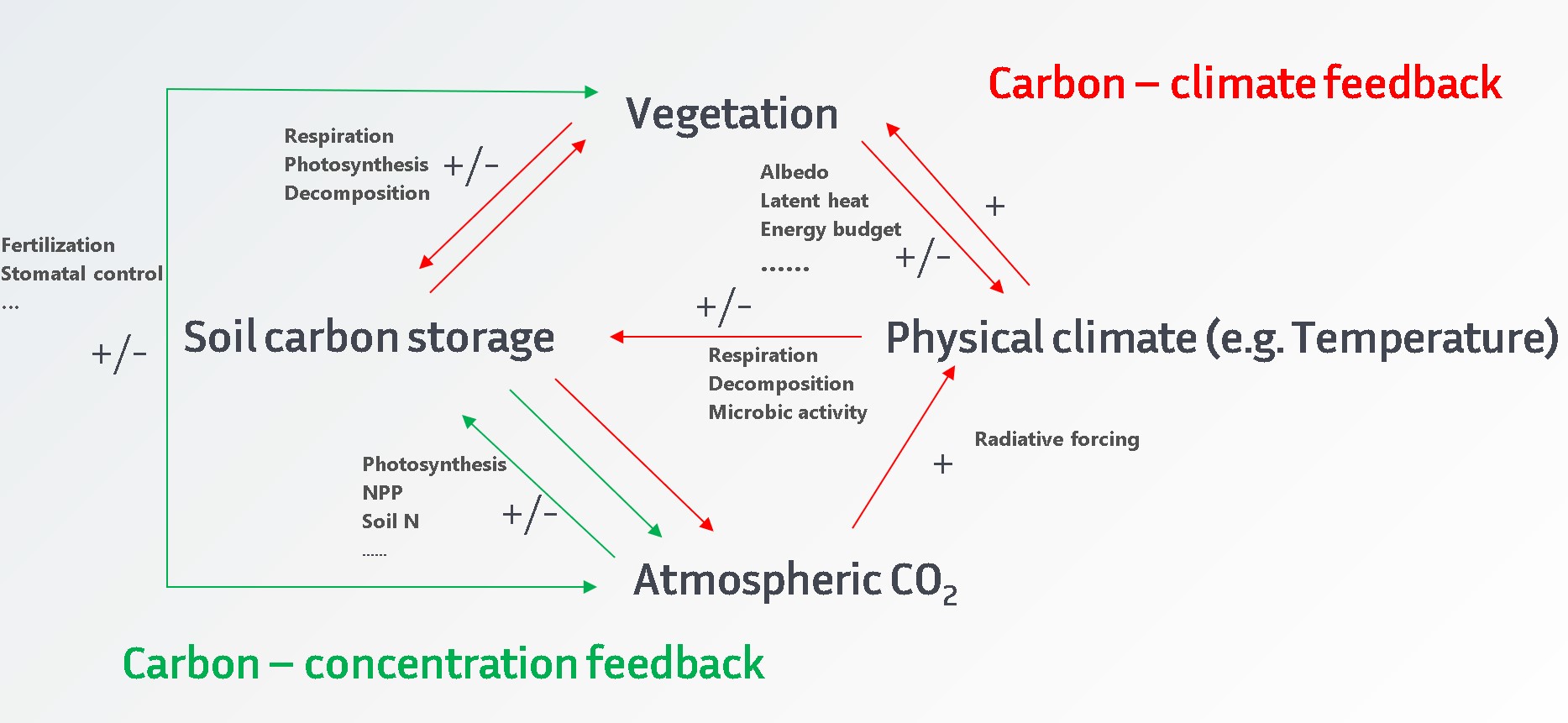